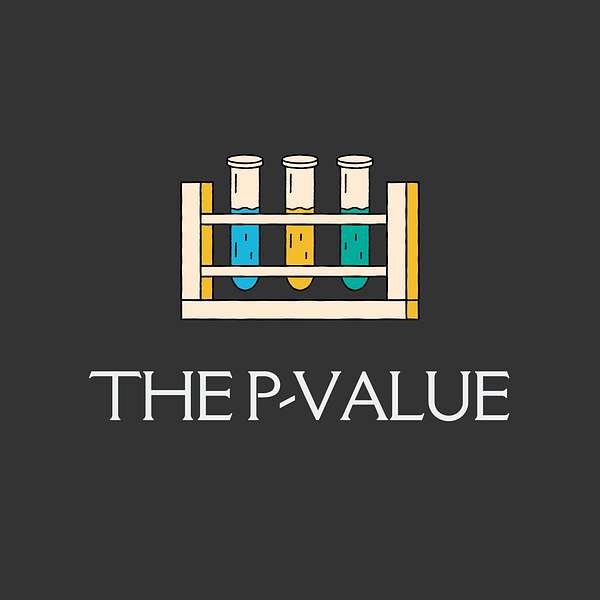
The P-Value Podcast
The P-Value Podcast
Inductive Risk: Why social and ethical values should influence which hypotheses scientists accept
Prior to the arrival of Dutch explorers in in Western Australia in the 1690s, black swans were like unicorns in European thinking — an impossibility. Indeed, as early as Roman times black swans were offered up as the paradigmatic example of something impossible, every swan observed by Europeans to that point having been white. The inference from the observation “all observed swans are white” to the general conclusion “all swans are white” being made by Europeans, whilst ultimately mistaken, reflects a form reasoning common in science known as induction.
Science relies heavily on inductive reasoning. It proceeds largely by drawing a generalised conclusions about unobserved cases, from a limited set of observations. For example, when we carry out an experiment in the lab, we expect to see the sorts of regularities that hold there, also hold in the world. More concretely, when we carry out a large-scale human drug trial or set of trials, when moving to use the drug (or decide it’s not worth using) we infer that the patterns of effectiveness seen in the experimental context will be seen in real world cases.
MUSIC
Hello and welcome to the p-value. I am your host Dr Rachael Brown and in this episode of the p-value we are talking about inductive risk and why some philosophers of science think that our social and political values should influence which hypotheses scientists accept at least some of the time.
MUSIC
As the black swan example so starkly illustrates, whilst inductive reasoning is powerful, it doesn’t necessarily lead to true conclusions. The pre-1690s European naturalists had every reason to expect that they were right in their conclusion that all swans are white given their observations, but unbeknownst to them they were observing a biased subset of all the swans on Earth. Unfortunately, in science too we can never be sure we are not in a black swan kind of case and thus we can never be 100% certain of the truth of any conclusion we come to via induction. This is because we can never be sure that the observed instances, we use to draw a more general conclusion are not biased in some way. Is, for example, the set of human subjects that take part in a drug trial a representative sample of the broader population? Or is it a skewed subset? Even more concerning perhaps is the possibility that, even if we are looking at a representative subset of broader society, by pure bad luck alone we nonetheless end up with a set of results that do not reflect the true features of the world. Just like we can get by bad luck 100 heads in a row tossing a fair coin, the non-deterministic nature of many of the things we care about in science mean we can even with a good test end up with unrepresentative results and thus erroneous inductive conclusions.
Whilst a stark illustration, the black swan case is not an outlier. The history of science abounds with hypotheses or theories that have been believed to be true, only to later be overturned on the grounds of new evidence. That this is the case is a good sign, not a challenge to science. It suggests that we are not falling foul of the inductive gap between our evidence and our theory. It would be far more concerning if we never overturned our scientific theories than that we are testing them and refining them. In addition, there are various things scientists do to try to limit the chance we are making erroneous inductive generalisations, such as carrying out replication studies, using large sample sizes and testing generalisations in different conditions.
MUSIC
We often hear our politicians and policy-makers extol the virtues of simply “following the science”, the expectation being that science or the scientific consensus gives us a clear guide to public policy. Unfortunately, in virtue of the inherent challenges that induction presents means that the story here is rarely that simple. The move from scientific data to evidence based public policy is complex and inherently risky. Philosophers have dubbed this sort of risk “inductive risk”. It is the risk of erroneously accepting or rejecting hypotheses on the basis false negatives and false positive results.
Importantly, inductive risk is not a purely theoretical concern. Because of the practical implications that science has on our everyday lives, inductive failures can have very real societal implications. For example, when we infer from the success of a drug trial to the broader efficacy of a drug we make an inference which will have impacts for human health and wellbeing. If our evidence has falsely indicated that our drug is effective —a false positive finding— then patients may be prescribed an ineffective drug thereby incurring any side effects without benefits along with ongoing suffering. They also incur the opportunity costs of not taking some other drug that is effective. This can have dire consequences for some diseases. In the converse, however, if we get a false negative result, we can end up discarding a potentially beneficial treatment as effective.
It is tempting to think that the internal epistemic or constitutive values of science —those associated with acquiring true claims such as simplicity and accuracy — can solve the problem of induction. They should be able to tell us what the appropriate evidential threshold for accepting or rejecting a hypothesis is. Unfortunately, as US philosopher Helen Douglas, points out this is not so. Whilst these epistemic or constitutive values can help us assess how strong our evidence is for a theory or claim, they can’t tell us whether the evidence is strong enough to make a claim at a particular point in time. This might seem strange but think again about the drug trial case, whilst we can do a statistical analysis of our data get a p-value or probability value of less than 0.05 which tells us that there is a less than one in twenty chance that our results showing the drug is effective are due to chance, our analysis doesn’t tell us whether this should be the threshold for acceptance of the theory that the drug is effective.
Indeed, whilst the threshold for statistical significance is often given as a p-value of less than 0.05 in stats classes, different fields actually have different conventions regarding what is the required level of statistical significance for acceptance. Where the threshold is set by weighing up the costs of erroneously accepting or rejecting hypotheses on the basis of false negative and false positive results and the costs of getting more fine-grained data. Indeed, in some fields of physics the standard for statistical significance is far higher than in most biological sciences, requiring p values less than or equal to 0.001, in part because of the sort of phenomena being studied and the ability to get very clean results. Importantly, here however, it is not epistemic values or constitutive values that are doing the work here but more human contextual values relating to our tolerance for risk relative to our pragmatic, social and ethical interests. Yes, we can set our standard for evidence at a p-value of less than 0.05 simply by conventional fiat but this would be arbitrary. The only non-arbitrary way of establishing what counts as sufficient evidence for accepting a theory is to consider the context of use for the information being generated by science.
If we accept these arguments, then that contextual social and ethical values are an unavoidable part of science, particularly our practices of theory acceptance. Heather Douglas argues forcefully that not only do values influence science in this way, but that they should and furthermore that scientists have a responsibility to transparently use values in their scientific practice. Let’s turn to this latter argument now.
MUSIC
The pandemic has highlighted the central role played by science and scientists in guiding societal decision making worldwide. It is this role that has motivated Douglas, amongst others going back to Richard Rudner in the 50s, to argue that scientists have a special responsibility when it comes to what threshold they have for acceptance or rejecting a hypothesis.
When any member of society makes a public claim they bear some responsibility for considering the consequences of that claim. Someone yelling “fire” in a cinema without due justification for thinking there really was a fire would be considered reckless and irresponsible. Similarly, someone not yelling “fire” until it was licking at their toes would also be considered irresponsible for being overly cautious. Scientists, as members of society bear this same sort of responsibility, when making public claims about theories and their acceptance or rejection. Failing to warn the public about a possible health risk despite good evidence is considered irresponsible. Similarly giving a warning of a health risk without due evidence is also considered reprehensible. Many philosophers of science argue that scientists bear an even greater responsibility in this domain than the general public because of the special role that science and scientists play in society. Scientists are recognised as experts in their fields and seen as an authority in the eyes of the public and public policy makers and this carries with it a responsibility or duty of care. Consider for example the controversy surrounding the announcement of the 2020 COVID-19 pandemic and around the public health interventions in the ensuring months. There has been a huge amount of pressure on scientists to be right all the time and a great deal of acrimony directed at those considerd to have been gungho in accepting or rejecting hypotheses alongside those who have been considered too cautious and thus to have delayed implementing useful public health interventions.
This stuff can be really high stakes and, as already outlined, and Douglas, Rudner and others argue that science itself doesn’t give us the tools to solve this problem. Purely epistemic reasons do not give us the threshold for acceptance and thus there is no (responsible) way to set the trade off between false positives and false negatives other than by appeal to the non-epistemic costs associated with acting on different types of error. In some cases, this will be not so challenging. Those working on the origins of the universe for example, can typically have a very high standard for theory acceptance because waiting for more data bears no social costs. Those working on a vaccine during the early days of a pandemic are not so lucky. Waiting for a very high standard of evidence costs human lives, but so too does implementing an ineffective vaccine or one that results in unnecessarily large numbers of vaccine related deaths or injuries. It is thus, our social and ethical values which should decide our evidential standards in these cases and it is totally acceptable, indeed necessary, for them to do so.
This seems like a very reasonable move but it involves a rather counter intuitive result. Effectively it is saying that our social and ethical values should determine which scientific theories we accept or not. It appears to go against everything we typically hold to be important in science – the so-called value-free and objective picture of science.
MUSIC
For some philosophers, those taking Rudner and Douglas’ sort of line and arguing for a central and unavoidable role for social and ethical values in theory acceptance have gone too far. Ernan McMullin and Richard Jeffrey, for example, argue that we can’t expect that scientists consider the social and ethical consequences of erroneously accepting or rejecting a theory. To do so is to impinge on the integrity of science and the central importance of impartiality and disinterestedness in good scientific practice. Rather they say, scientists need not solve problems of inductive risk at all they should simply report epistemic probabilities. In effect, they should just ditch the idea of there being a conventional threshold of acceptance. It is this convention that is the problem, and it is easily got rid of. This seems too simple, however. Scientists don’t just do analyses and report results, they offer interpretations of those results, an interpretation that requires specific knowledge and expertise that only scientists have.
One reply to this objection is to simply say that Douglas, Rudner et al. are not making a claim about what should happen in science, they are making a claim about what is happening already to some degree. All they are doing is pointing it out and making it transparent. This seems like a fair response. That the acceptance threshold of p is less than or equal to 0.05 is often not understood to be a mere convention by working scientists, public policy makers and the lay public alike is a problem. Moreover, it is a problem that is only made more pressing by the potential costs that can be incurred when we make bad decisions about appropriate thresholds. In this light Douglas, Rudner et al. are pushing for a major re-think not only about theory acceptance but how we view science altogether.
MUSIC